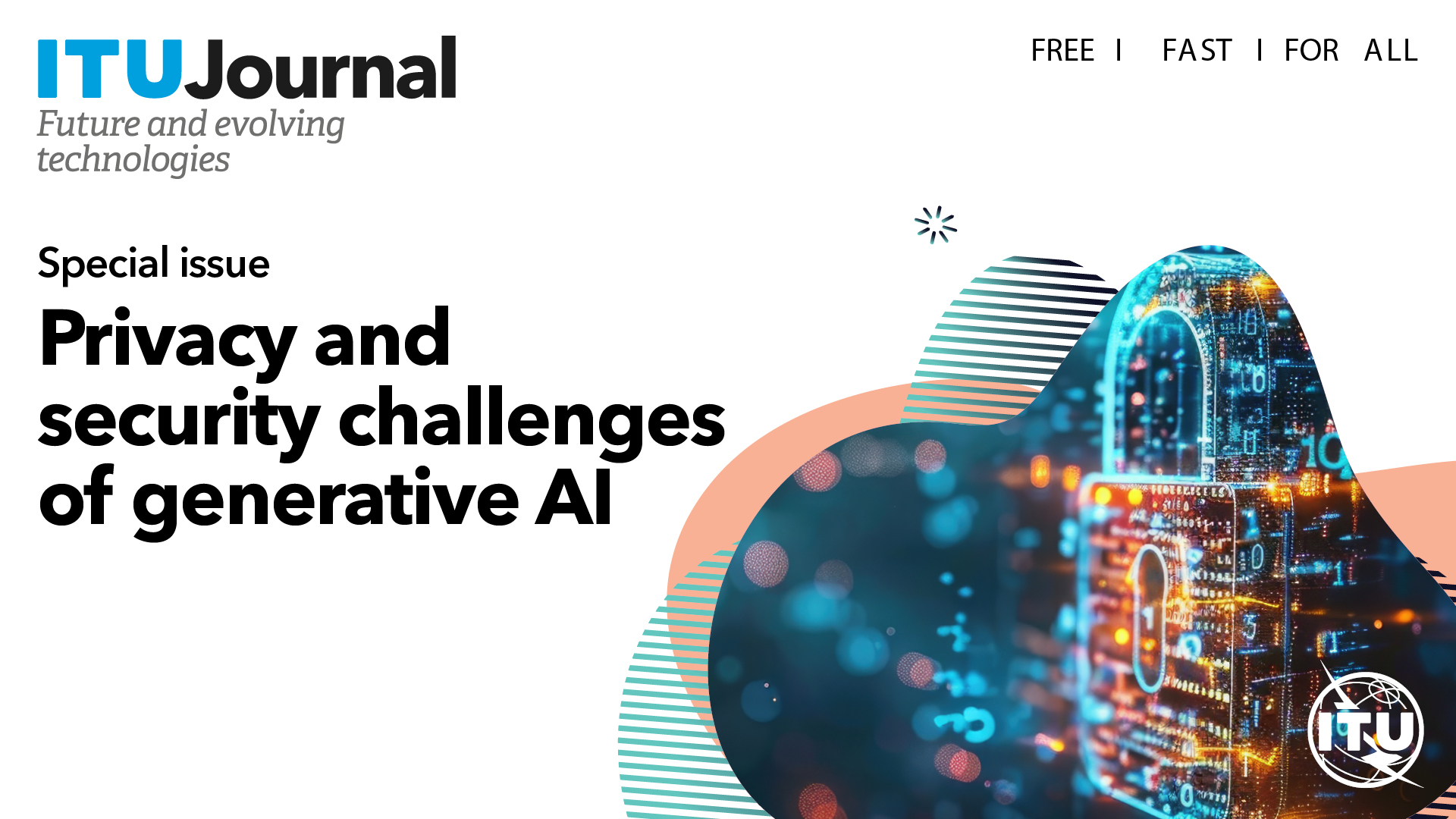
Theme
The use of Large Language Model (LLM) and Generative Pre-trained Transforms (GPT) technology based on Generative Artificial Intelligence (GAI) has become ubiquitous across various industries and societal domains due to their powerful capabilities in extracting, processing and expanding data, information, and knowledge. GAI can address the escalating demands of our digital life, encompassing cost, power, capacity, coverage, latency, efficiency, flexibility, compatibility, quality of experience and services.
However, as GAI application systems proliferate, privacy and security concerns have assumed an increasing pivotal role in their rapid development and massive deployment. Private and secure generative AI technology not only prevents unauthorized data and model parameters usage but also safeguards highly sensitive, proprietary, classified or private information during both training and inference phases. This adherence to security standards and privacy laws, such as the European GDPR rules or the US HIPPA rules, is crucial.
Fully Homomorphic Encryption (FHE) technology emerges as the most promising solution to address privacy and security concerns in GAI. Unlike GAI operating in plaintext formats, FHE based on GAI conducts all computations and operations in encrypted ciphertext formats. However, this comes with a substantial increase in implementation complexity— on the order of 1,000 times compared to plaintext formats. Consequently, this imposes great limitations and challenges on processing architecture, memory access, computational capability, inference latency, data interfaces and bandwidths of hardware and silicon convergence for FHE-based GAI. Realizing secure and private GAI is a very challenging task and requires significant efforts from the related industry, research, and regulatory authorities for success.
This special issue aims to catalyze and steer the advancement of novel and improved systems to enable private and secure Generative AI, by fostering collaboration among scientists, engineers, broadcasters, manufacturers, software developers, and other related professionals.
Keywords
Fully Homomorphic Encryption (FHE), information security, data privacy, machine learning, neural networks, Generative Pre-trained Transforms (GPT), Large Language Model (LLM), Generative Artificial Intelligence (GAI), learning and inference, fine-tuning, transfer learning, attention and query
Suggest topics (but not limited to)
Algorithms, architectures and applications:
- Encryption and decryption for private and secure GAI
- Pipelining, parallel and distributed processing with co-design of algorithm and hardware
- Ciphertexts-data driven programming platform and models
- New computing architecture, memory access and data-interface
- FHE based training and inference in GAI
Deployment, standardization and development:
- Standardization, technical regulations and specifications for GAI
- Secure multiple-party computation, differential privacy and federal learning
- FHE based development libraries and open source software
Information and signal processing:
- Learning with noise, bootstrapping and programming bootstrapping
- Private LLM with fine-tuning, transfer learning and lower-rank adaption
- FHE based transforms and neural networks
Download the FULL call for papers here.
Leading Guest Editor
Guest Editors
Rosario Cammarota, Intel Labs, USA
|
Paul Master, Cornami, USA
|
Nir Drucke, IBM-Europe, Israel
|
Donghoon Yoo, Desilo, Korea
|
Konstantinos Plataniotis, University of Toronto, Canada
|